Get the full backstory of the algorithm’s evolution and how BERT has improved human language understanding for machines.
If you have been keeping an eye on Twitter SEO over the past week you’ll have likely noticed an uptick in the number of gifs and images featuring the character Bert (and sometimes Ernie) from Sesame Street.
This is because, last week Google announced an imminent algorithmic update would be rolling out, impacting 10% of queries in search results, and also affect featured snippet results in countries where they were present; which is not trivial.
The update is named Google BERT (Hence the Sesame Street connection – and the gifs).
Google describes BERT as the largest change to its search system since the company introduced RankBrain, almost five years ago, and probably one of the largest changes in search ever.
The news of BERT’s arrival and its impending impact has caused a stir in the SEO community, along with some confusion as to what BERT does, and what it means for the industry overall.
With this in mind, let’s take a look at what BERT is, BERT’s background, the need for BERT and the challenges it aims to resolve, the current situation (i.e. what it means for SEO), and where things might be headed.
What is BERT?
BERT is a technologically ground-breaking natural language processing model/framework which has taken the machine learning world by storm since its release as an academic research paper. The research paper is entitled BERT: Pre-training of Deep Bidirectional Transformers for Language Understanding (Devlin et al, 2018).
Following paper publication Google AI Research team announced BERT as an open source contribution.
A year later, Google announced a Google BERT algorithmic update rolling out in production search. Google linked the BERT algorithmic update to the BERT research paper, emphasizing BERT’s importance for contextual language understanding in content and queries, and therefore intent, particularly for conversational search.
So, just what is BERT really?
BERT is described as a pre-trained deep learning natural language framework that has given state-of-the-art results on a wide variety of natural language processing tasks. Whilst in the research stages, and prior to being added to production search systems, BERT achieved state-of-the-art results on 11 different natural language processing tasks. These natural language processing tasks include, amongst others, sentiment analysis, named entity determination, textual entailment (aka next sentence prediction), semantic role labeling, text classification and coreference resolution. BERT also helps with the disambiguation of words with multiple meanings known as polysemous words, in context.
BERT is referred to as a model in many articles, however, it is more of a framework, since it provides the basis for machine learning practitioners to build their own fine-tuned BERT-like versions to meet a wealth of different tasks, and this is likely how Google is implementing it too.
BERT was originally pre-trained on the whole of the English Wikipedia and Brown Corpus and is fine-tuned on downstream natural language processing tasks like question and answering sentence pairs. So, it is not so much a one-time algorithmic change, but rather a fundamental layer which seeks to help with understanding and disambiguating the linguistic nuances in sentences and phrases, continually fine-tuning itself and adjusting to improve.
The BERT backstory
To begin to realize the value BERT brings we need to take a look at prior developments.
The natural language challenge
Understanding the way words fit together with structure and meaning is a field of study connected to linguistics. Natural language understanding (NLU), or NLP, as it is otherwise known, dates back over 60 years, to the original Turing Test paper and definitions of what constitutes AI, and possibly earlier.
This compelling field faces unsolved problems, many relating to the ambiguous nature of language (lexical ambiguity). Almost every other word in the English language has multiple meanings.
These challenges naturally extend to a web of ever-increasing content as search engines try to interpret intent to meet informational needs expressed by users in written and spoken queries.
Lexical ambiguity
In linguistics, ambiguity is at the sentence rather than word level. Words with multiple meanings combine to make ambiguous sentences and phrases become increasingly difficult to understand.
According to Stephen Clark, formerly of Cambridge University, and now a full-time research scientist at Deepmind:
“Ambiguity is the greatest bottleneck to computational knowledge acquisition, the killer problem of all natural language processing.”
In the example below, taken from WordNet (a lexical database which groups English words into synsets (sets of synonyms)), we see the word “bass” has multiple meanings, with several relating to music and tone, and some relating to fish.
Furthermore, the word “bass” in a musical context can be both a noun part-of-speech or an adjective part-of-speech, confusing matters further.
Noun
- S: (n) bass (the lowest part of the musical range)
- S: (n) bass, bass part (the lowest part in polyphonic music)
- S: (n) bass, basso (an adult male singer with the lowest voice)
- S: (n) sea bass, bass (the lean flesh of a saltwater fish of the family Serranidae)
- S: (n) freshwater bass, bass (any of various North American freshwater fish with lean flesh (especially of the genus Micropterus))
- S: (n) bass, bass voice, basso (the lowest adult male singing voice)
- S: (n) bass (the member with the lowest range of a family of musical instruments)
- S: (n) bass (nontechnical name for any of numerous edible marine and freshwater spiny-finned fishes)
Adjective
- S: (adj) bass, deep (having or denoting a low vocal or instrumental range) “a deep voice”; “a bass voice is lower than a baritone voice”; “a bass clarinet”
Polysemy and homonymy
Words with multiple meanings are considered polysemous or homonymous.
Polysemy
Polysemous words are words with two or more meanings, with roots in the same origin, and are extremely subtle and nuanced. The verb ‘get’, a polysemous word, for example, could mean ‘to procure’,’ to acquire’, or ‘to understand’. Another verb, ‘run’ is polysemous and is the largest entry in the Oxford English Dictionary with 606 different meanings.
Homonymy
Homonyms are the other main type of word with multiple meanings, but homonyms are less nuanced than polysemous words since their meanings are often very different. For example, “rose,” which is a homonym, could mean to “rise up” or it could be a flower. These two-word meanings are not related at all.
Homographs and homophones
Types of homonyms can be even more granular too. ‘Rose’ and ‘Bass’ (from the earlier example), are considered homographs because they are spelled the same and have different meanings, whereas homophones are spelled differently, but sound the same. The English language is particularly problematic for homophones. You can find a list over over 400 English homophone examples here, but just a few examples of homophones include:
- Draft, draught
- Dual, duel
- Made, maid
- For, fore, four
- To, too, two
- There, their
- Where, wear, were
At a spoken phrase-level word when combined can suddenly become ambiguous phrases even when the words themselves are not homophones.
For example, the phrases “four candles” and “fork handles” when splitting into separate words have no confusing qualities and are not homophones, but when combined they sound almost identical.
Suddenly these spoken words could be confused as having the same meaning as each other whilst having entirely different meanings. Even humans can confuse the meaning of phrases like these since humans are not perfect after all. Hence, the many comedy shows feature “play on words” and linguistic nuances. These spoken nuances have the potential to be particularly problematic for conversational search.
Synonymy is different
To clarify, synonyms are different from polysemy and homonymy, since synonymous words mean the same as each other (or very similar), but are different words.
An example of synonymous words would be the adjectives “tiny,” “little” and “mini” as synonyms of “small.”
Coreference resolution
Pronouns like “they,” “he,” “it,” “them,” “she” can be a troublesome challenge too in natural language understanding, and even more so, third-person pronouns, since it is easy to lose track of who is being referred to in sentences and paragraphs. The language challenge presented by pronouns is referred to as coreference resolution, with particular nuances of coreference resolution being an anaphoric or cataphoric resolution.
You can consider this simply “being able to keep track” of what, or who, is being talked about, or written about, but here the challenge is explained further.
Anaphora and cataphora resolution
Anaphora resolution is the problem of trying to tie mentions of items as pronouns or noun phrases from earlier in a piece of text (such as people, places, things). Cataphora resolution, which is less common than anaphora resolution, is the challenge of understanding what is being referred to as a pronoun or noun phrase before the “thing” (person, place, thing) is mentioned later in a sentence or phrase.
Here is an example of anaphoric resolution:
“John helped Mary. He was kind.”
Where “he” is the pronoun (anaphora) to resolve back to “John.”
And another:
The car is falling apart, but it still works.
Here is an example of cataphora, which also contains anaphora too:
“She was at NYU when Mary realized she had lost her keys.”
The first “she” in the example above is cataphora because it relates to Mary who has not yet been mentioned in the sentence. The second “she” is an anaphora since that “she” relates also to Mary, who has been mentioned previously in the sentence.
Multi-sentential resolution
As phrases and sentences combine referring to people, places and things (entities) as pronouns, these references become increasingly complicated to separate. This is particularly so if multiple entities resolve to begin to be added to the text, as well as the growing number of sentences.
Here is an example from this Cornell explanation of coreference resolution and anaphora:
a) John took two trips around France.
b) They were both wonderful.
Humans and ambiguity
Although imperfect, humans are mostly unconcerned by these lexical challenges of coreference resolution and polysemy since we have a notion of common-sense understanding.
We understand what “she” or “they” refer to when reading multiple sentences and paragraphs or hearing back and forth conversation since we can keep track of who is the subject focus of attention.
We automatically realize, for example, when a sentence contains other related words, like “deposit,” or “cheque / check” and “cash,” since this all relates to “bank” as a financial institute, rather than a river “bank.”
In order words, we are aware of the context within which the words and sentences are uttered or written; and it makes sense to us. We are therefore able to deal with ambiguity and nuance relatively easily.
Machines and ambiguity
Machines do not automatically understand the contextual word connections needed to disambiguate “bank” (river) and “bank” (financial institute). Even less so, polysemous words with nuanced multiple meanings, like “get” and “run.” Machines lose track of who is being spoken about in sentences easily as well, so coreference resolution is a major challenge too.
When the spoken word such as conversational search (and homophones), enters the mix, all of these become even more difficult, particularly when you start to add sentences and phrases together.
How search engines learn language
So just how have linguists and search engine researchers enabling machines to understand the disambiguated meaning of words, sentences and phrases in natural language?
“Wouldn’t it be nice if Google understood the meaning of your phrase, rather than just the words that are in the phrase?” said Google’s Eric Schmidt back in March 2009, just before the company announced rolling out their first semantic offerings.
This signaled one of the first moves away from “strings to things,” and is perhaps the advent of entity-oriented search implementation by Google.
One of the products mentioned in Eric Schmidt’s post was ‘related things’ displayed in search results pages. An example of “angular momentum,” “special relativity,” “big bang” and “quantum mechanic” as related items, was provided.
These items could be considered co-occurring items that live near each other in natural language through ‘relatedness’. The connections are relatively loose but you might expect to find them co-existing in web page content together.
So how do search engines map these “related things” together?
Co-occurrence and distributional similarity
In computational linguistics, co-occurrence holds true the idea that words with similar meanings or related words tend to live very near each other in natural language. In other words, they tend to be in close proximity in sentences and paragraphs or bodies of text overall (sometimes referred to as corpora).
This field of studying word relationships and co-occurrence is called Firthian Linguistics, and its roots are usually connected with 1950s linguist John Firth, who famously said:
“You shall know a word by the company it keeps.”
(Firth, J.R. 1957)
Similarity and relatedness
In Firthian linguistics, words and concepts living together in nearby spaces in text are either similar or related.
Words which are similar “types of things” are thought to have semantic similarity. This is based upon measures of distance between “isA” concepts which are concepts that are types of a “thing.” For example, a car and a bus have semantic similarity because they are both types of vehicles. Both car and bus could fill the gap in a sentence such as:
“A ____ is a vehicle,” since both cars and buses are vehicles.
Relatedness is different from semantic similarity. Relatedness is considered ‘distributional similarity’ since words related to isA entities can provide clear cues as to what the entity is.
For example, a car is similar to a bus since they are both vehicles, but a car is related to concepts of “road” and “driving.”
You might expect to find a car mentioned in amongst a page about road and driving, or in a page sitting nearby (linked or in the section – category or subcategory) a page about a car.
This is a very good video on the notions of similarity and relatedness as scaffolding for natural language.
Humans naturally understand this co-occurrence as part of common sense understanding, and it was used in the example mentioned earlier around “bank” (river) and “bank” (financial institute).
Content around a bank topic as a financial institute will likely contain words about the topic of finance, rather than the topic of rivers, or fishing, or be linked to a page about finance.
Therefore, “bank’s” company are “finance,” “cash,” “cheque” and so forth.
Knowledge graphs and repositories
Whenever semantic search and entities are mentioned we probably think immediately of search engine knowledge graphs and structured data, but natural language understanding is not structured data.
However, structured data makes natural language understanding easier for search engines through disambiguation via distributional similarity since the ‘company’ of a word gives an indication as to topics in the content.
Connections between entities and their relations mapped to a knowledge graph and tied to unique concept ids are strong (e.g. schema and structured data).
Furthermore, some parts of entity understanding are made possible as a result of natural language processing, in the form of entity determination (deciding in a body of text which of two or more entities of the same name are being referred to), since entity recognition is not automatically unambiguous.
Mention of the word “Mozart” in a piece of text might well mean “Mozart,” the composer, “Mozart” cafe, “Mozart” street, and there are umpteen people and places with the same name as each other.
The majority of the web is not structured at all. When considering the whole web, even semi-structured data such as semantic headings, bullet and numbered lists and tabular data make up only a very small part of it. There are lots of gaps of loose ambiguous text in sentences, phrases and paragraphs.
Natural language processing is about understanding the loose unstructured text in sentences, phrases and paragraphs between all of those “things” which are “known of” (the entities). A form of “gap filling” in the hot mess between entities. Similarity and relatedness, and distributional similarity) help with this.
Relatedness can be weak or strong
Whilst data connections between the nodes and edges of entities and their relations are strong, the similarity is arguably weaker, and relatedness weaker still. Relatedness may even be considered vague.
The similarity connection between apples and pears as “isA” things is stronger than a relatedness connection of “peel,” “eat,” “core” to apple, since this could easily be another fruit which is peeled and with a core.
An apple is not really identified as being a clear “thing” here simply by seeing the words “peel,” “eat” and “core.” However, relatedness does provide hints to narrow down the types of “things” nearby in content.
Computational linguistics
Much “gap filling” natural language research could be considered computational linguistics; a field that combines maths, physics and language, particularly linear algebra and vectors and power laws.
Natural language and distributional frequencies overall have a number of unexplained phenomena (for example, the Zipf Mystery), and there are several papers about the “strangeness” of words and use of language.
On the whole, however, much of language can be resolved by mathematical computations around where words live together (the company they keep), and this forms a large part of how search engines are beginning to resolve natural language challenges (including the BERT update).
Word embeddings and co-occurrence vectors
Simply put, word embeddings are a mathematical way to identify and cluster in a mathematical space, words which “live” nearby each other in a real-world collection of text, otherwise known as a text corpus. For example, the book “War and Peace” is an example of a large text corpus, as is Wikipedia.
Word embeddings are merely mathematical representations of words that typically live near each other whenever they are found in a body of text, mapped to vectors (mathematical spaces) using real numbers.
These word embeddings take the notions of co-occurrence, relatedness and distributional similarity, with words simply mapped to their company and stored in co-occurrence vector spaces. The vector ‘numbers’ are then used by computational linguists across a wide range of natural language understanding tasks to try to teach machines how humans use language based on the words that live near each other.
WordSim353 Dataset examples
We know that approaches around similarity and relatedness with these co-occurrence vectors and word embeddings have been part of research by members of Google’s conversational search research team to learn word’s meaning.
For example, “A study on similarity and relatedness using distributional and WordNet-based approaches,” which utilizes the Wordsim353 Dataset to understand distributional similarity.
This type of similarity and relatedness in datasets is used to build out “word embeddings” mapped to mathematical spaces (vectors) in bodies of text.
Here is a very small example of words that commonly occur together in content from the Wordsim353 Dataset, which is downloadable as a Zip format for further exploration too. Provided by human graders, the score in the right-hand column is based on how similar the two words in the left-hand and middle columns are.
money | cash | 9.15 |
coast | shore | 9.1 |
money | cash | 9.08 |
money | currency | 9.04 |
football | soccer | 9.03 |
magician | wizard | 9.02 |
Word2Vec
Semi-supervised and unsupervised machine learning approaches are now part of this natural language learning process too, which has turbo-charged computational linguistics.
Neural nets are trained to understand the words that live near each other to gain similarity and relatedness measures and build word embeddings.
These are then used in more specific natural language understanding tasks to teach machines how humans understand language.
A popular tool to create these mathematical co-occurrence vector spaces using text as input and vectors as output is Google’s Word2Vec. The output of Word2Vec can create a vector file that can be utilized on many different types of natural language processing tasks.
The two main Word2Vec machine learning methods are Skip-gram and Continuous Bag of Words.
The Skip-gram model predicts the words (context) around the target word (target), whereas the Continuous Bag of Words model predicts the target word from the words around the target (context).
These unsupervised learning models are fed word pairs through a moving “context window” with a number of words around a target word. The target word does not have to be in the center of the “context window” which is made up of a given number of surrounding words but can be to the left or right side of the context window.
An important point to note is moving context windows are uni-directional. I.e. the window moves over the words in only one direction, from either left to right or right to left.
Part-of-speech tagging
Another important part of computational linguistics designed to teach neural nets human language concerns mapping words in training documents to different parts-of-speech. These parts of speech include the likes of nouns, adjectives, verbs and pronouns.
Linguists have extended the many parts-of-speech to be increasingly fine-grained too, going well beyond common parts of speech we all know of, such as nouns, verbs and adjectives, These extended parts of speech include the likes of VBP (Verb, non-3rd person singular present), VBZ (Verb, 3rd person singular present) and PRP$ (Possessive pronoun).
Word’s meaning in part-of-speech form can be tagged up as parts of speech using a number of taggers with a varying granularity of word’s meaning, for example, The Penn Treebank Tagger has 36 different parts of speech tags and the CLAWS7 part of speech tagger has a whopping 146 different parts of speech tags.
Google Pygmalion, for example, which is Google’s team of linguists, who work on conversational search and assistant, used part of speech tagging as part of training neural nets for answer generation in featured snippets and sentence compression.
Understanding parts-of-speech in a given sentence allows machines to begin to gain an understanding of how human language works, particularly for the purposes of conversational search, and conversational context.
To illustrate, we can see from the example “Part of Speech” tagger below, the sentence:
“Search Engine Land is an online search industry news publication.”
This is tagged as “Noun / noun / noun / verb / determiner / adjective / noun / noun / noun / noun” when highlighted as different parts of speech.

Problems with language learning methods
Despite all of the progress search engines and computational linguists had made, unsupervised and semi-supervised approaches like Word2Vec and Google Pygmalion have a number of shortcomings preventing scaled human language understanding.
It is easy to see how these were certainly holding back progress in conversational search.
Pygmalion is unscalable for internationalization
Labeling training datasets with parts-of-speech tagged annotations can be both time-consuming and expensive for any organization. Furthermore, humans are not perfect and there is room for error and disagreement. The part of speech a particular word belongs to in a given context can keep linguists debating amongst themselves for hours.
Google’s team of linguists (Google Pygmalion) working on Google Assistant, for example, in 2016 was made up of around 100 Ph.D. linguists. In an interview with Wired Magazine, Google Product Manager, David Orr explained how the company still needed its team of Ph.D. linguists who label parts of speech (referring to this as the ‘gold’ data), in ways that help neural nets understand how human language works.
Orr said of Pygmalion:
“The team spans between 20 and 30 languages. But the hope is that companies like Google can eventually move to a more automated form of AI called ‘unsupervised learning.’”
By 2019, the Pygmalion team was an army of 200 linguists around the globe made up of a mixture of both permanent and agency staff, but was not without its challenges due to the laborious and disheartening nature of manual tagging work, and the long hours involved.
In the same Wired article, Chris Nicholson, who is the founder of a deep learning company called Skymind commented about the un-scaleable nature of projects like Google Pygmalion, particularly from an internationalisation perspective, since part of speech tagging would need to be carried out by linguists across all the languages of the world to be truly multilingual.
Internationalization of conversational search
The manual tagging involved in Pygmalion does not appear to take into consideration any transferable natural phenomenons of computational linguistics. For example, Zipfs Law, a distributional frequency power law, dictates that in any given language the distributional frequency of a word is proportional to one over its rank, and this holds true even for languages not yet translated.
Uni-directional nature of ‘context windows’ in RNNs (Recurrent Neural Networks)
Training models in the likes of Skip-gram and Continuous Bag of Words are Uni-Directional in that the context-window containing the target word and the context words around it to the left and to the right only go in one direction. The words after the target word are not yet seen so the whole context of the sentence is incomplete until the very last word, which carries the risk of some contextual patterns being missed.
A good example is provided of the challenge of uni-directional moving context-windows by Jacob Uszkoreit on the Google AI blog when talking about the transformer architecture.
Deciding on the most likely meaning and appropriate representation of the word “bank” in the sentence: “I arrived at the bank after crossing the…” requires knowing if the sentence ends in “… road.” or “… river.”
 100vw, 800px” data-lazy-src=”https://searchengineland.com/figz/wp-content/seloads/2019/11/image10-800×433.png” /></figure>
<h2>Text cohesion missing</h2>
<p>The uni-directional training approaches prevent the presence of text cohesion.</p>
<p>Like Ludwig Wittgenstein, a philosopher famously said in 1953:</p>
<blockquote class=)
“The meaning of a word is its use in the language.”
(Wittgenstein, 1953)
Often the tiny words and the way words are held together are the ‘glue’ which bring common sense in language. This ‘glue’ overall is called ‘text cohesion’. It’s the combination of entities and the different parts-of-speech around them formulated together in a particular order which makes a sentence have structure and meaning. The order in which a word sits in a sentence or phrase too also adds to this context.
Without this contextual glue of these surrounding words in the right order, the word itself simply has no meaning.
The meaning of the same word can change too as a sentence or phrase develops due to dependencies on co-existing sentence or phrase members, changing context with it.
Furthermore, linguists may disagree over which particular part-of-speech in a given context a word belongs to in the first place.
Let us take the example word “bucket.” As humans we can automatically visualize a bucket that can be filled with water as a “thing,” but there are nuances everywhere.
What if the word bucket word were in the sentence “He kicked the bucket,” or “I have yet to cross that off my bucket list?” Suddenly the word takes on a whole new meaning. Without the text-cohesion of the accompanying and often tiny words around “bucket” we cannot know whether bucket refers to a water-carrying implement or a list of life goals.
Word embeddings are context-free
The word embedding model provided by the likes of Word2Vec knows the words somehow live together but does not understand in what context they should be used. True context is only possible when all of the words in a sentence are taken into consideration. For example, Word2Vec does not know when river (bank) is the right context, or bank (deposit). Whilst later models such as ELMo trained on both the left side and right side of a target word, these were carried out separately rather than looking at all of the words (to the left and the right) simultaneously, and still did not provide true context.
Polysemy and homonymy handled incorrectly
Word embeddings like Word2Vec do not handle polysemy and homonyms correctly. As a single word with multiple meanings is mapped to just one single vector. Therefore there is a need to disambiguate further. We know there are many words with the same meaning (for example, ‘run’ with 606 different meanings), so this was a shortcoming. As illustrated earlier polysemy is particularly problematic since polysemous words have the same root origins and are extremely nuanced.
Coreference resolution still problematic
Search engines were still struggling with the challenging problem of anaphora and cataphora resolution, which was particularly problematic for conversational search and assistant which may have back and forth multi-turn questions and answers.
Being able to track which entities are being referred to is critical for these types of spoken queries.
Shortage of training data
Modern deep learning-based NLP models learn best when they are trained on huge amounts of annotated training examples, and a lack of training data was a common problem holding back the research field overall.
So, how does BERT help improve search engine language understanding?
With these short-comings above in mind, how has BERT helped search engines (and other researchers) to understand language?
What makes BERT so special?
There are several elements that make BERT so special for search and beyond (the World – yes, it is that big as a research foundation for natural language processing). Several of the special features can be found in BERT’s paper title – BERT: Bi-directional Encoder Representations from Transformers.
B – Bi-Directional
E – Encoder
R – Representations
T – Transformers
But there are other exciting developments BERT brings to the field of natural language understanding too.
These include:
- Pre-training from unlabelled text
- Bi-directional contextual models
- The use of a transformer architecture
- Masked language modeling
- Focused attention
- Textual entailment (next sentence prediction)
- Disambiguation through context open-sourced
Pre-training from unlabeled text
The ‘magic’ of BERT is its implementation of bi-directional training on an unlabelled corpus of text since for many years in the field of natural language understanding, text collections had been manually tagged up by teams of linguists assigning various parts of speech to each word.
BERT was the first natural language framework/architecture to be pre-trained using unsupervised learning on pure plain text (2.5 billion words+ from English Wikipedia) rather than labeled corpora.
Prior models had required manual labeling and the building of distributed representations of words (word embeddings and word vectors), or needed part of speech taggers to identify the different types of words present in a body of text. These past approaches are similar to the tagging we mentioned earlier by Google Pygmalion.
BERT learns language from understanding text cohesion from this large body of content in plain text and is then educated further by fine-tuning on smaller, more specific natural language tasks. BERT also self-learns over time too.
Bi-directional contextual models
BERT is the first deeply bi-directional natural language model, but what does this mean?
Bi-directional and uni-directional modeling
True contextual understanding comes from being able to see all the words in a sentence at the same time and understand how all of the words impact the context of the other words in the sentence too.
The part of speech a particular word belongs to can literally change as the sentence develops.
For example, although unlikely to be a query, if we take a spoken sentence which might well appear in natural conversation (albeit rarely):
“I like how you like that he likes that.”
as the sentence develops the part of speech which the word “like” relates to as the context builds around each mention of the word changes so that the word “like,” although textually is the same word, contextually is different parts of speech dependent upon its place in the sentence or phrase.
Past natural language training models were trained in a uni-directional manner. Word’s meaning in a context window moved along from either left to right or right to left with a given number of words around the target word (the word’s context or “it’s company”). This meant words not yet seen in context cannot be taken into consideration in a sentence and they might actually change the meaning of other words in natural language. Uni-directional moving context windows, therefore, have the potential to miss some important changing contexts.
For example, in the sentence:
“Dawn, how are you?”
The word “are” might be the target word and the left context of “are” is “Dawn, how.” The right context of the word is “you.”
BERT is able to look at both sides of a target word and the whole sentence simultaneously in the way that humans look at the whole context of a sentence rather than looking at only a part of it. The whole sentence, both left and right of a target word can be considered in the context simultaneously.
Transformers / Transformer architecture
Most tasks in natural language understanding are built on probability predictions. What is the likelihood that this sentence relates to the next sentence, or what is the likelihood that this word is part of that sentence? BERT’s architecture and masked language modeling prediction systems are partly designed to identify ambiguous words that change the meanings of sentences and phrases and identify the correct one. Learnings are carried forward increasingly by BERT’s systems.
The Transformer uses fixation on words in the context of all of the other words in sentences or phrases without which the sentence could be ambiguous.
This fixated attention comes from a paper called ‘Attention is all you need’ (Vaswani et al, 2017), published a year earlier than the BERT research paper, with the transformer application then built into the BERT research.
Essentially, BERT is able to look at all the context in text-cohesion by focusing attention on a given word in a sentence whilst also identifying all of the context of the other words in relation to the word. This is achieved simultaneously using transformers combined with bi-directional pre-training.
This helps with a number of long-standing linguistic challenges for natural language understanding, including coreference resolution. This is because entities can be focused on in a sentence as a target word and their pronouns or the noun-phrases referencing them resolved back to the entity or entities in the sentence or phrase.
In this way the concepts and context of who, or what, a particular sentence is relating to specifically, is not lost along the way.
Furthermore, the focused attention also helps with the disambiguation of polysemous words and homonyms by utilizing a probability prediction / weight based on the whole context of the word in context with all of the other words in the sentence. The other words are given a weighted attention score to indicate how much each adds to the context of the target word as a representation of “meaning.” Words in a sentence about the “bank” which add strong disambiguating context such as “deposit” would be given more weight in a sentence about the “bank” (financial institute) to resolve the representational context to that of a financial institute.
The encoder representations part of the BERT name is part of the transformer architecture. The encoder is the sentence input translated to representations of words meaning and the decoder is the processed text output in a contextualized form.
In the image below we can see that ‘it’ is strongly being connected with “the” and “animal” to resolve back the reference to “the animal” as “it” as a resolution of anaphora.
 100vw, 458px” data-lazy-src=”https://searchengineland.com/figz/wp-content/seloads/2019/11/image5.png” /></figure>
<p>This fixation also helps with the changing “part of speech” a word’s order in a sentence could have since we know that the same word can be different parts of speech depending upon its context.</p>
<p>The example provided by Google below illustrates the importance of different parts of speech and word category disambiguation. Whilst a tiny word, the word ‘to’ here changes the meaning of the query altogether once it is taken into consideration in the full context of the phrase or sentence.</p>
<figure class=)

Masked Language Modelling (MLM Training)
Also known as “the Cloze Procedure,” which has been around for a very long time. The BERT architecture analyzes sentences with some words randomly masked out and attempts to correctly predict what the “hidden” word is.
The purpose of this is to prevent target words in the training process passing through the BERT transformer architecture from inadvertently seeing themselves during bi-directional training when all of the words are looked at together for combined context. Ie. it avoids a type of erroneous infinite loop in natural language machine learning, which would skew word’s meaning.
Textual entailment (next sentence prediction)
One of the major innovations of BERT is that it is supposed to be able to predict what you’re going to say next, or as the New York Times phrased it in Oct 2018, “Finally, a machine that can finish your sentences.”
BERT is trained to predict from pairs of sentences whether the second sentence provided is the right fit from a corpus of text.
NB: It seems this feature during the past year was deemed as unreliable in the original BERT model and other open-source offerings have been built to resolve this weakness. Google’s ALBERT resolves this issue.
Textual entailment is a type of “what comes next?” in a body of text. In addition to textual entailment, the concept is also known as ‘next sentence prediction’. Textual entailment is a natural language processing task involving pairs of sentences. The first sentence is analyzed and then a level of confidence determined to predict whether a given second hypothesized sentence in the pair “fits” logically as the suitable next sentence, or not, with either a positive, negative, or neutral prediction, from a text collection under scrutiny.
Three examples from Wikipedia of each type of textual entailment prediction (neutral / positive / negative) are below. Textual Entailment Examples (Source: Wikipedia)
An example of a positive TE (text entails hypothesis) is:
text: If you help the needy, God will reward you.
hypothesis: Giving money to a poor man has good consequences.
An example of a negative TE (text contradicts hypothesis) is:
text: If you help the needy, God will reward you.
hypothesis: Giving money to a poor man has no consequences.
An example of a non-TE (text does not entail nor contradict) is:
text: If you help the needy, God will reward you.
hypothesis: Giving money to a poor man will make you a better person.
Disambiguation breakthroughs from open-sourced contributions
BERT has not just appeared from thin air, and BERT is no ordinary algorithmic update either since BERT is also an open-source natural language understanding framework as well.
Ground-breaking “disambiguation from context empowered by open-sourced contributions,” could be used to summarise BERT’s main value add to natural language understanding. In addition to being the biggest change to Google’s search system in five years (or ever), BERT also represents probably the biggest leap forward in growing contextual understanding of natural language by computers of all time.
Whilst Google BERT may be new to the SEO world it is well known in the NLU world generally and has caused much excitement over the past 12 months. BERT has provided a hockey stick improvement across many types of natural language understanding tasks not just for Google, but a myriad of both industrial and academic researchers seeking to utilize language understanding in their work, and even commercial applications.
After the publication of the BERT research paper, Google announced they would be open-sourcing vanilla BERT. In the 12 months since publication alone, the original BERT paper has been cited in further research 1,997 times at the date of writing.
There are many different types of BERT models now in existence, going well beyond the confines of Google Search.
A search for Google BERT in Google Scholar returns hundreds of 2019 published research paper entries extending on BERT in a myriad of ways, with BERT now being used in all manner of research into natural language.
Research papers traverse an eclectic mix of language tasks, domain verticals (for example clinical fields), media types (video, images) and across multiple languages. BERT’s use cases are far-reaching, from identifying offensive tweets using BERT and SVMs to using BERT and CNNs for Russian Troll Detection on Reddit, to categorizing via prediction movies according to sentiment analysis from IMDB, or predicting the next sentence in a question and answer pair as part of a dataset.
Through this open-source approach, BERT goes a long way toward solving some long-standing linguistic problems in research, by simply providing a strong foundation to fine-tune from for anyone with a mind to do so. The codebase is downloadable from the Google Research Team’s Github page.
By providing Vanilla BERT as a great ‘starter for ten’ springboard for machine learning enthusiasts to build upon, Google has helped to push the boundaries of State of the art (SOTA) natural language understanding tasks. Vanilla BERT can be likened to a CMS plugins, theme, or module which provides a strong foundation for a particular functionality but can then be developed further. Another simpler similarity might be likening the pre-training and fine-tuning parts of BERT for machine learning engineers to buying an off-the-peg suit from a high street store then visiting a tailor to turn up the hems so it is fit for purpose at a more unique needs level.
As Vanilla BERT comes pre-trained (on Wikipedia and Brown corpus), researchers need only fine-tune their own models and additional parameters on top of the already trained model in just a few epochs (loops / iterations through the training model with the new fine-tuned elements included).
At the time of BERT’s October 2018, paper publication BERT beat state of the art (SOTA) benchmarks across 11 different types of natural language understanding tasks, including question and answering, sentiment analysis, named entity determination, sentiment classification and analysis, sentence pair-matching and natural language inference.
Furthermore, BERT may have started as the state-of-the-art natural language framework but very quickly other researchers, including some from other huge AI-focused companies such as Microsoft, IBM and Facebook, have taken BERT and extended upon it to produce their own record-beating open-source contributions. Subsequently, models other than BERT have become state of the art since BERT’s release.
Facebook’s Liu et al entered the BERTathon with their own version extending upon BERT – RoBERTa. claiming the original BERT was significantly undertrained and professing to have improved upon, and beaten, any other model versions of BERT up to that point.
Microsoft also beat the original BERT with MT-DNN, extending upon a model they proposed in 2015 but adding on the bi-directional pre-training architecture of BERT to improve further.

There are many other BERT-based models too, including Google’s own XLNet and ALBERT (Toyota and Google), IBM’s BERT-mtl, and even now Google T5 emerging.
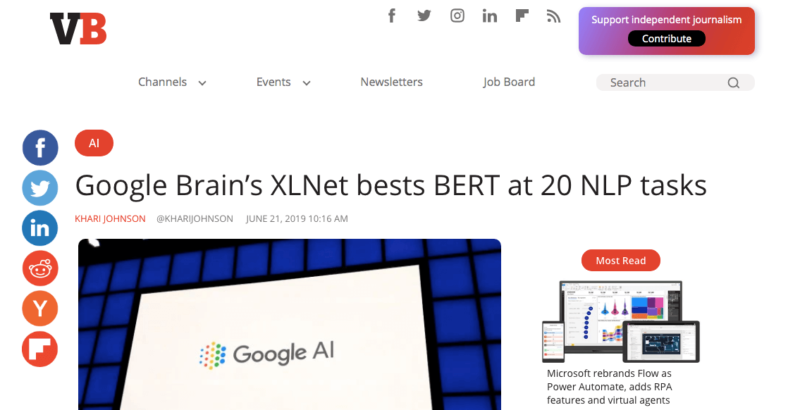
The field is fiercely competitive and NLU machine learning engineer teams compete with both each other and non-expert human understanding benchmarks on public leaderboards, adding an element of gamification to the field.
Amongst the most popular leaderboards are the very competitive SQuAD, and GLUE.
SQuAD stands for The Stanford Question and Answering Dataset which is built from questions based on Wikipedia articles with answers provided by crowdworkers.
The current SQuAD 2.0 version of the dataset is the second iteration created because SQuAD 1.1 was all but beaten by natural language researchers. The second-generation dataset, SQuAD 2.0 represented a harder dataset of questions, and also contained an intentional number of adversarial questions in the dataset (questions for which there was no answer). The logic behind this adversarial question inclusion is intentional and designed to train models to learn to know what they do not know (i.e an unanswerable question).
GLUE is the General Language Understanding Evaluation dataset and leaderboard. SuperGLUE is the second generation of GLUE created because GLUE again became too easy for machine learning models to beat.

Most of the public leaderboards across the machine learning field double up as academic papers accompanied by rich question and answer datasets for competitors to fine-tune their models on. MS MARCO, for example, is an academic paper, dataset and accompanying leaderboard published by Microsoft; AKA Microsoft MAchine Reading COmprehension Dataset.
The MSMARCO dataset is made up of over a million real Bing user queries and over 180,000 natural language answers. Any researchers can utilize this dataset to fine-tune models.
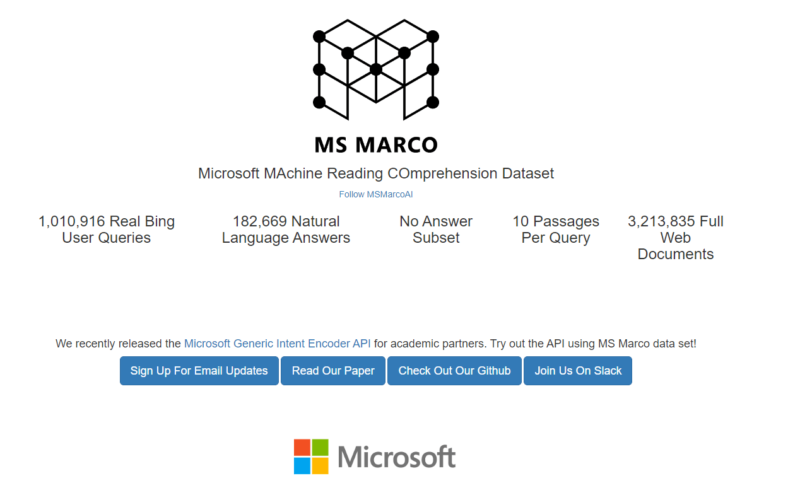
Efficiency and computational expense
Late 2018 through 2019 can be remembered as a year of furious public leaderboard leap-frogging to create the current state of the art natural language machine learning model.
As the race to reach the top of the various state of the art leaderboards heated up, so too did the size of the model’s machine learning engineers built and the number of parameters added based on the belief that more data increases the likelihood for more accuracy. However as model sizes grew so did the size of resources needed for fine-tuning and further training, which was clearly an unsustainable open-source path.
Victor Sanh, of Hugging Face (an organization seeking to promote the continuing democracy of AI) writes, on the subject of the drastically increasing sizes of new models:
“The latest model from Nvidia has 8.3 billion parameters: 24 times larger than BERT-large, 5 times larger than GPT-2, while RoBERTa, the latest work from Facebook AI, was trained on 160GB of text 😵”
To illustrate the original BERT sizes – BERT-Base and BERT-Large, with 3 times the number of parameters of BERT-Base.
BERT–Base, Cased : 12-layer, 768-hidden, 12-heads , 110M parameters. BERT–Large, Cased : 24-layer, 1024-hidden, 16-heads, 340M parameters.
Escalating costs and data sizes meant some more efficient, less computationally and financially expensive models needed to be built.
Welcome Google ALBERT, Hugging Face DistilBERT and FastBERT
Google’s ALBERT, was released in September 2019 and is a joint work between Google AI and Toyota’s research team. ALBERT is considered BERT’s natural successor since it also achieves state of the art scores across a number of natural language processing tasks but is able to achieve these in a much more efficient and less computationally expensive manner.
Large ALBERT has 18 times fewer parameters than BERT-Large. One of the main standout innovations with ALBERT over BERT is also a fix of a next-sentence prediction task which proved to be unreliable as BERT came under scrutiny in the open-source space throughout the course of the year.
We can see here at the time of writing, on SQuAD 2.0 that ALBERT is the current SOTA model leading the way. ALBERT is faster and leaner than the original BERT and also achieves State of the Art (SOTA) on a number of natural language processing tasks.

Other efficiency and budget focused, scaled-down BERT type models recently introduced are DistilBERT, purporting to be smaller, lighter, cheaper and faster, and FastBERT.
So, what does BERT mean for SEO?
BERT may be known among SEOs as an algorithmic update, but in reality, it is more “the application” of a multi-layer system that understands polysemous nuance and is better able to resolve co-references about “things” in natural language continually fine-tuning through self-learning.
The whole purpose of BERT is to improve human language understanding for machines. In a search perspective this could be in written or spoken queries issued by search engine users, and in the content search engines gather and index. BERT in search is mostly about resolving linguistic ambiguity in natural language. BERT provides text-cohesion which comes from often the small details in a sentence that provides structure and meaning.
BERT is not an algorithmic update like Penguin or Panda since BERT does not judge web pages either negatively or positively, but more improves the understanding of human language for Google search. As a result, Google understands much more about the meaning of content on pages it comes across and also the queries users issue taking word’s full context into consideration.
 100vw, 691px” data-lazy-src=”https://searchengineland.com/figz/wp-content/seloads/2019/11/image6.png” /></figure>
<h2>BERT is about sentences and phrases</h2>
<p>Ambiguity is not at a word level, but at a sentence level, since it is about the combination of words with multiple meanings which cause ambiguity.</p>
<figure class=)

BERT helps with polysemic resolution
Google BERT helps Google search to understand “text-cohesion” and disambiguate in phrases and sentences, particularly where polysemic nuances could change the contextual meaning of words.
In particular, the nuance of polysemous words and homonyms with multiple meanings, such as ‘to’, ‘two’, ‘to’, and ‘stand’ and ‘stand’, as provided in the Google examples, illustrate the nuance which had previously been missed, or misinterpreted, in search.
Ambiguous and nuanced queries impacted
The 10% of search queries which BERT will impact may be very nuanced ones impacted by the improved contextual glue of text cohesion and disambiguation. Furthermore, this might well impact understanding even more of the 15% of new queries which Google sees every day, many of which relate to real-world events and burstiness / temporal queries rather than simply long-tailed queries.
Recall and precision impacted (impressions?)
Precision in ambiguous query meeting will likely be greatly improved which may mean query expansion and relaxation to include more results (recall) may be reduced.
Precision is a measure of result quality, whereas recall simply relates to return any pages which may be relevant to a query.
We may see this reduction in recall reflected in the number of impressions we see in Google Search Console, particularly for pages with long-form content which might currently be in recall for queries they are not particularly relevant for.
BERT will help with coreference resolution
BERT(the research paper and language model)’s capabilities with coreference resolution means the Google algorithm likely helps Google Search to keep track of entities when pronouns and noun-phrases refer to them.
BERT’s attention mechanism is able to focus on the entity under focus and resolve all references in sentences and phrases back to that using a probability determination / score.
Pronouns of “he,” “she,” “they,” “it” and so forth will be much easier for Google to map back in both content and queries, spoken and in written text.
This may be particularly important for longer paragraphs with multiple entities referenced in text for featured snippet generation and voice search answer extraction / conversational search.
BERT serves a multitude of purposes
Google BERT is probably what could be considered a Swiss army knife type of tool for Google Search.
BERT provides a solid linguistic foundation for Google search to continually tweak and adjust weights and parameters since there are many different types of natural language understanding tasks that could be undertaken.
Tasks may include:
- Coreference resolution (keeping track of who, or what, a sentence or phrase refers to in context or an extensive conversational query)
- Polysemy resolution (dealing with ambiguous nuance)
- Homonym resolution (dealing with understanding words which sound the same, but mean different things
- Named entity determination (understanding which, from a number of named entities, text relates to since named entity recognition is not named entity determination or disambiguation), or one of many other tasks.
- Textual entailment (next sentence prediction)
BERT will be huge for conversational search and assistant
Expect a quantum leap forward in terms of relevance matching to conversational search as Google’s in-practice model continues to teach itself with more queries and sentence pairs.
It’s likely these quantum leaps will not just be in the English language, but very soon, in international languages too since there is a feed-forward learning element within BERT that seems to transfer to other languages.
BERT will likely help Google to scale conversational search
Expect over the short to medium term a quantum leap forward in application to voice search however since the heavy lifting of building out the language understanding held back by Pygmalion’s manual process could be no more.
The earlier referenced 2016 Wired article concluded with a definition of AI automated, unsupervised learning which might replace Google Pygmalion and create a scalable approach to train neural nets:
“This is when machines learn from unlabeled data – massive amounts of digital information culled from the internet and other sources.”
(Wired, 2016)
This sounds like Google BERT.
We also know featured snippets were being created by Pygmalion too.
While it is unclear whether BERT will have an impact on Pygmalion’s presence and workload, nor if featured snippets will be generated in the same way as previously, Google has announced BERT will be used for featured snippets and is pre-trained on purely a large text corpus.
Furthermore, the self-learning nature of a BERT type foundation continually fed queries and retrieving responses and featured snippets will naturally pass the learnings forward and become even more fine-tuned.
BERT, therefore, could provide a potentially, hugely scalable alternative to the laborious work of Pygmalion.
International SEO may benefit dramatically too
One of the major impacts of BERT could be in the area of international search since the learnings BERT picks up in one language seem to have some transferable value to other languages and domains too.
Out of the box, BERT appears to have some multi-lingual properties somehow derived from a monolingual (single language understanding) corpora and then extended to 104 languages, in the form of M-BERT (Multilingual BERT).
A paper by Pires, Schlinger & Garrette tested the multilingual capabilities of Multilingual BERT and found that it “surprisingly good at zero-shot cross-lingual model transfer.” (Pires, Schlinger & Garrette, 2019). This is almost akin to being able to understand a language you have never seen before since zero-shot learning aims to help machines categorize objects that they have never seen before.
Questions and answers
Question and answering directly in SERPs will likely continue to get more accurate which could lead to a further reduction in click through to sites.
In the same way MSMARCO is used for fine-tuning and is a real dataset of human questions and answers from Bing users, Google will likely continue to fine-tune its model in real-life search over time through real user human queries and answers feeding forward learnings.
As language continues to be understood paraphrase understanding improved by Google BERT might also impact related queries in “People Also Ask.”
Textual entailment (next sentence prediction)
The back and forth of conversational search, and multi-turn question and answering for assistant will also likely benefit considerably from BERT’s ‘textual entailment’ (next sentence prediction) feature, particularly the ability to predict “what comes next” in a query exchange scenario. However, this might not seem apparent as quickly as some of the initial BERT impacts.
Furthermore, since BERT can understand different meanings for the same things in sentences, aligning queries formulated in one way and resolving them to answers which amount to the same thing will be much easier.
I asked Dr. Mohammad Aliannejadi about the value BERT provides for conversational search research. Dr. Aliannejadi is an information retrieval researcher who recently defended his Ph.D. research work on conversational search, supervised by Professor Fabio Crestani, one of the authors of “Mobile information retrieval.”
Part of Dr. Aliannejadi’s research work explored the effects of asking clarifying questions for conversational assistants, and utilized BERT within its methodology.
Dr. Aliannejadi spoke of BERT’s value:
“BERT represents the whole sentence, and so it is representing the context of the sentence and can model the semantic relationship between two sentences. The other powerful feature is the ability to fine-tune it in just a few epochs. So, you have a general tool and then make it specific to your problem.”
Named entity determination
One of the natural language processing tasks undertaken by the likes of a fine-tuned BERT model could be entity determination. Entity determination is deciding the probability that a particular named entity is being referred to from more than one choice of named entity with the same name.
Named entity recognition is not named entity disambiguation nor named entity determination.
In an AMA on Reddit Google’s Gary Illyes confirmed that unlinked mentions of brand names can be used for this named entity determination purpose currently.
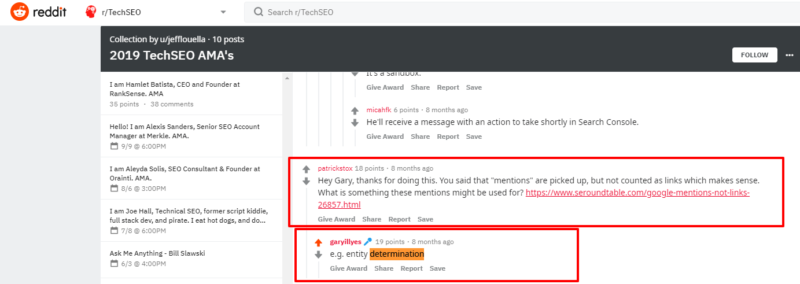
BERT will assist with understanding when a named entity is recognized but could be one of a number of named entities with the same name as each other.
An example of multiple named entities with the same name is in the example below. Whilst these entities may be recognized by their name they need to be disambiguated one from the other. Potentially an area BERT can help with.
We can see from a search in Wikipedia below the word “Harris” returns many named entities called “Harris.”

BERT could be BERT by name, but not by nature
It is not clear whether the Google BERT update uses the original BERT or the much leaner and inexpensive ALBERT, or another hybrid variant of the many models now available, but since ALBERT can be fine-tuned with far fewer parameters than BERT this might make sense.
This could well mean the algorithm BERT in practice may not look very much at all like the original BERT in the first published paper, but a more recent improved version which looks much more like the (also open-sourced) engineering efforts of others aiming to build the latest SOTA models.
BERT may be a completely re-engineered large scale production version, or a more computationally inexpensive and improved version of BERT, such as the joint work of Toyota and Google, ALBERT.
Furthermore, BERT may continue to evolve into other models since Google T5 Team also now has a model on the public SuperGLUE leaderboards called simply T5.
BERT may be BERT in name, but not in nature.
Can you optimize your SEO for BERT?
Probably not.
The inner workings of BERT are complex and multi-layered. So much so, there is now even a field of study called “Bertology” which has been created by the team at Hugging Face.
It is highly unlikely any search engineer questioned could explain the reasons why something like BERT would make the decisions it does with regards to rankings (or anything).
Furthermore, since BERT can be fine-tuned across parameters and multiple weights then self-learns in an unsupervised feed-forward fashion, in a continual loop, it is considered a black-box algorithm. A form of unexplainable AI.
BERT is thought to not always know why it makes decisions itself. How are SEOs then expected to try to “optimize” for it?
BERT is designed to understand natural language so keep it natural.
We should continue to create compelling, engaging, informative and well-structured content and website architectures in the same way you would write, and build sites, for humans.
The improvements are on the search engine side of things and are a positive rather than a negative.
Google simply got better at understanding the contextual glue provided by text cohesion in sentences and phrases combined and will become increasingly better at understanding the nuances as BERT self-learns.
Search engines still have a long way to go
Search engines still have a long way to go and BERT is only a part of that improvement along the way, particularly since a word’s context is not the same as search engine user’s context, or sequential informational needs which are infinitely more challenging problems.
SEOs still have a lot of work to do to help search engine users find their way and help to meet the right informational need at the right time.